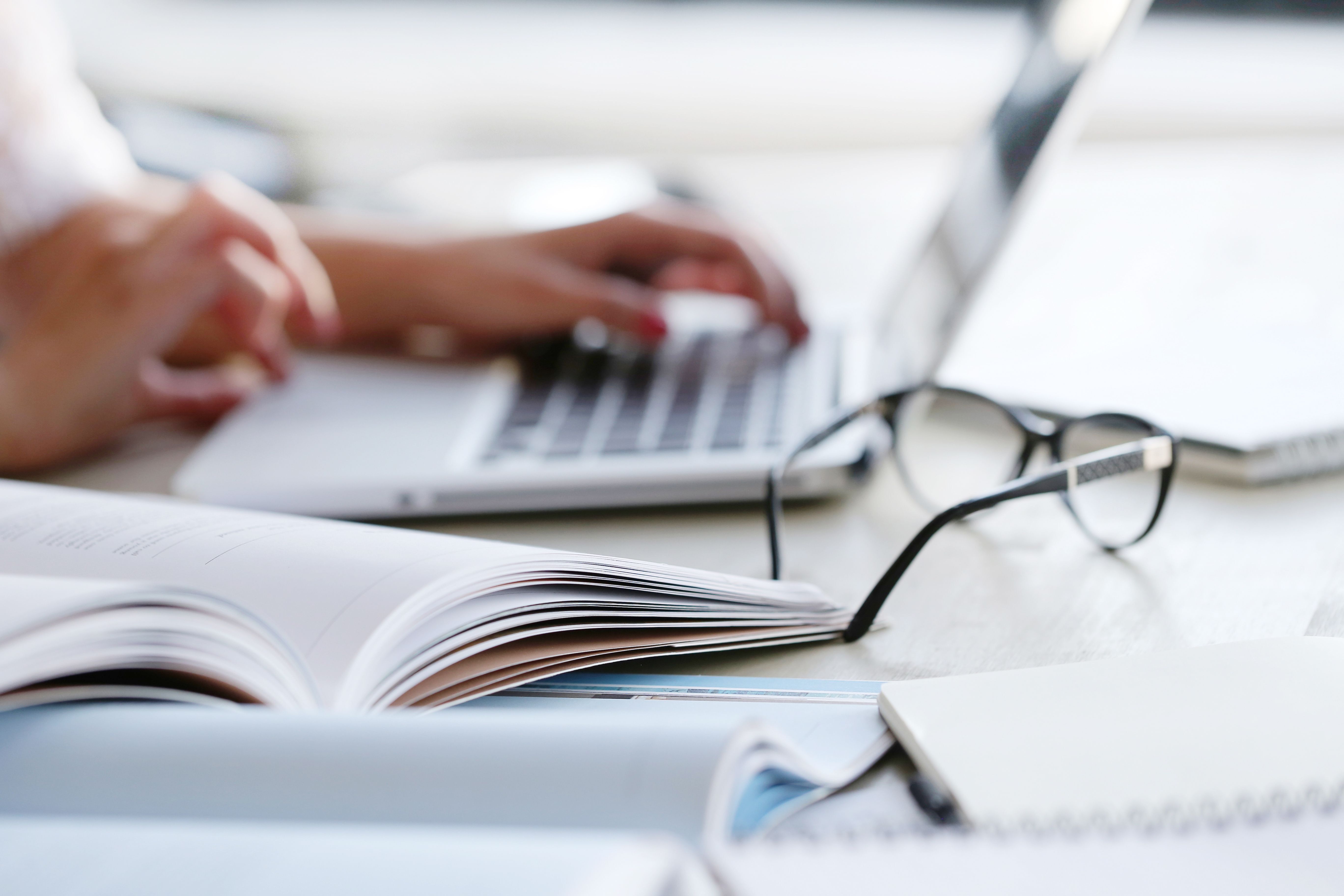
Can electronic health records help to predict more individuals at risk for out-of-hospital cardiac arrest? New research says yes.
In a study published this month, researchers from the University of Washington (UW) used machine learning technology to determine whether electronic health record (EHR) data could help to identify cardiac arrest in the general population and to determine which factors contribute most to the life-threatening condition.
(Note: for this blog, out-of-hospital cardiac arrest is referred to as cardiac arrest.)
While cardiac arrest risk is commonly linked to heart-related conditions such as coronary artery disease and high blood pressure, researchers identified new factors – such as marital status and substance use disorder – that may also contribute to the life-threatening event.
With the aid of machine learning – a type of technology where computers are taught to learn from data – researchers compared the health records of about 2,300 patients who experienced a cardiac arrest to those of more than 26,600 patients, matched by sex and age, who hadn’t. Patients captured in the study sought care at University of Washington Medicine (UW Medicine), a large academic health system serving the Seattle area.
The results identified several differences in patients who had a cardiac arrest and those who hadn’t, including some that could serve as new predictors of cardiac arrest. Here are four of the differences.
1. Social and demographic
People with cardiac arrest where less likely to be white, to be married, and to have private health insurance.
2. Vital signs
People with cardiac arrest had higher heart rate, breathing rate, and blood pressure. They also had less oxygen in their blood.
3. Heart function
People with cardiac arrest had several differences in heart function, including longer time needed for the heart to go through one complete cycle of beating and resting.
4. Non-heart-related conditions
People with cardiac arrest had fluid and electrolyte disorders, and alcohol and substance abuse disorders.
Prior studies, centered primarily in white populations, have suggested that coronary artery disease is responsible for cardiac arrest in 75%-80% of patients overall. However, in the UW study, only 28% of individuals with cardiac arrest had coronary disease. This could mean that non-heart factors, such as alcohol and substance abuse, may be as important in triggering cardiac arrest.
Improved prediction could lead to better prevention
Researchers adjusted the machine learning model to learn how well it could identify cardiac arrest risk if deployed across 1.5 million patients in the UW Medicine system. They determined it could correctly predict cardiac arrest in 1-2 people per 100 per year who had risk factors included in the expanded model. While 1 to 2% accuracy may seem low, it’s far better than the current 1 in 1,000 prediction accuracy based on cardiovascular risk factors alone.
Researchers at UW called for public health strategies that reflect the complexity of cardiac arrest, while noting that machine learning that incorporates health record data from the general population may serve as an effective screening tool for cardiac arrest.
Improved prediction could lead to be better intervention and prevention. A primary care doctor might identify a specific patient's risk factors and collaborate with them to implement lifestyle changes, thereby reducing the likelihood of a cardiac arrest.
At Starting Hearts, we can provide specific training for making a life-saving difference.
Contact us for more information: info@startinghearts.org.
Patrick Golden is a healthcare writer based in Massachusetts